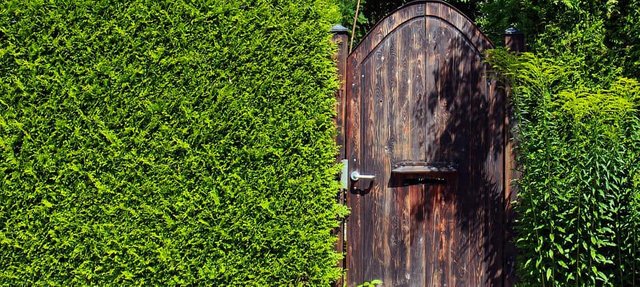
1.
두 번 JP Morgan이 발표한 기계학습과 관련한 논문 혹은 보고서를 소개했습니다.
JP Morgan이 발행한 기계학습 및 빅데이타 안내서
Morgan Stanley와 JP Morgan이 연구하는 RL
오늘은 JP Morgan의 Hans Bühler이 발표한 Deep Hedging: Hedging Derivatives Under Generic Market Frictions Using Reinforcement Learning을 소개합니다. 2018년 1월에 발표한 논문입니다. Deep Hedging을 소개한 기사입니다.
So the JP Morgan quants tried something different. Instead of feeding the machine a model, they let it formulate its own hedging strategy. An artificial neural network was trained to identify patterns and relationships from historical data. It then used a technique called reinforcement learning to refine its strategies based on simulated trades.The result of the experiment will come as no surprise to anyone that has been following recent advances in artificial intelligence. The so-called ‘deep hedging’ strategies developed by the machine outperformed existing ones based on classical models.
Last year, JP Morgan began using the self-taught algorithms to hedge some of its vanilla index options portfolios. The bank now plans to roll out similar technology for hedging single stocks, baskets and light exotics.
Deep hedging feels like the start of something big in quantitative finance. Several other firms, including Bank of America and Societe Generale, are working on similar projects. Some quants are already talking about a future of “model-free pricing”. But the machines are not infallible. Their performance is heavily reliant on the data used to train them, and errors in the training data, no matter how small, can make the resulting strategies very unstable. And even a well-trained machine cannot generalise or extrapolate beyond its training data, so it must be retrained every time there is a structural change in the markets.
The other problem is interpretability. Machines are not very transparent. Neural networks have complex structures and comb through millions of data points, which makes it hard to pinpoint how they come up with answers, or why something went wrong. To operate these machines safely, humans will have to learn new skills. “People who today spend their time adjusting for the deficiencies in classic Greek-type models now need to understand how the statistics work,” Hans Buehler, global head of equities analytics, automation and optimisation at JP Morgan, said in a recent Risk.net podcast.
The machines are coming for your pricing models중에서
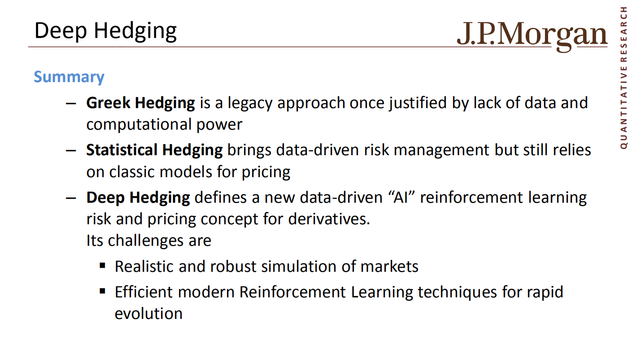
위 발표문을 보시면 아시겠지만 3세대 Hedging입니다.
[gview file="http://smallake.kr/wp-content/uploads/2019/10/SSRN-id3355706.pdf"]
[gview file="http://smallake.kr/wp-content/uploads/2019/10/1802.03042.pdf"]
[gview file="http://smallake.kr/wp-content/uploads/2019/10/Buehler-Swissquote2018.pdf"]
[gview file="http://smallake.kr/wp-content/uploads/2019/10/Deep-Hedging-Frontiers-Imperial-2.1.pdf"]
이상의 이론을 업무에 어떻게 적용할 수 있을까요? 이와 관련하여 발표한 자료가 Deep Hedging: from theory to practice입니다. 자료를 보시면 Deep Hedging을 설명하고 마지막에서 Market Dynamics(Generative Adversarial Market Dynamics)를 다루고 있습니다.
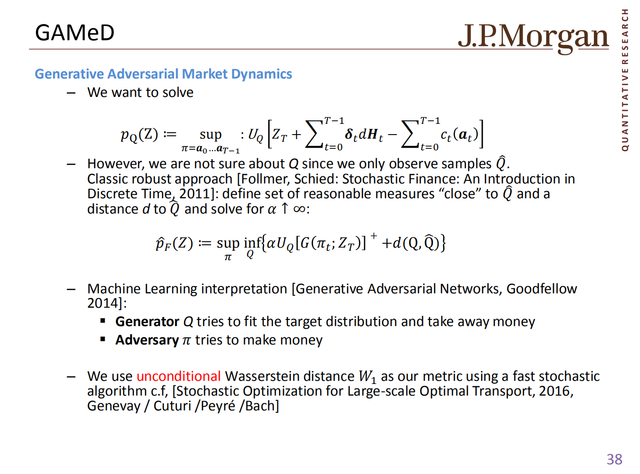
Deep Hedging을 실제 운용에 적용한 결과는 어떨까요? Derivatives JP Morgan turns to machine learning for options hedging중 일부입니다. JP Morgan의 경험과 Beacon Platform의 조사결과를 보면 비용을 절감하였다고 합니다.
JP Morgan is using machine learning to automate the hedging of some equity options, a move that one quant calls a “game-changer”.The bank started using machine learning to hedge a portion of its index vanilla flow book last year. Since then, it has been able to hedge its exposures faster, and quote higher volumes as a result.
“The real advantage is we are able to increase volumes quoted – because we are faster,” says Hans Buehler, global head of equities analytics, automation and optimisation at JP Morgan in London. “If you have to manually manage this, you have to divert somebody’s time and sit them down to focus on it.”
One senior quant calls JP Morgan’s approach a “base-level rethink” of hedging, which he says will benefit illiquid markets in particular. He estimates the technique has the potential to cut hedging costs for certain commodity derivatives by as much as 80%.
“There are lots of places in the market where there is either illiquidity in the hedging instruments you have or large transaction costs or products that have risks that are unhedgeable,” says Mark Higgins, chief operating officer and co-founder of Beacon Platform in New York and co-head of JP Morgan’s quantitative research team until 2014.
“In those places, it will be a real paradigm change in how people can approach optimal hedging,” he says.
It will be a real paradigm change in how people can approach optimal hedging
Mark Higgins, Beacon Platform
JP Morgan’s new hedging programme uses complex statistical regression, a type of machine learning that tries to find statistical relationships between variables by trawling through large amounts of data. The technique relies on historical market data rather than risk sensitivities – or Greeks – to estimate hedging costs, a dramatic shift from the popular Black-Scholes model.“Black-Scholes Greeks were very useful in the 1980s because we didn’t have a ton of data and we didn’t have a ton of computing power. So this was an approximation that worked very well for a long time. Today, we have much more data. If you revisit the problem of hedging derivatives now, I don’t think you would sit there and build the Black-Scholes model,” says Buehler.
Buehler was one of the co-authors of a recently published paper on so-called deep hedging. He says the contents of the paper are part of an “ambitious project” at the bank, which will allow it to hedge positions multiple time-steps ahead. This means it can provide hedges along a path of times rather than just a single period of time in the future.The research has already attracted interest from others in the industry who are eager to apply it in their own businesses. Beacon Platform, for instance, is researching the application of deep hedging in commodity derivatives and variable annuities.
For example, an investor that buys the rights to run a natural gas storage facility would need to determine the best way to delta-hedge their exposure to commodity prices. They could hedge at the storage location, where transaction costs might be high, or take on some basis risk and hedge at a more liquid hub location. Deep hedging techniques can tell the investor how to distribute their hedges between these locations.
“Deep hedging for that situation gives you a quantitative model that, when you actually introduce basis volatility and transaction costs at different locations, tells you interesting things about how you spread the delta-hedges in different locations and gives you a better business outcome,” says Higgins.
The same holds true for variable annuities sold by life insurance companies, which provide a variable payout at retirement based on the investments of the buyer. These products carry hard-to-hedge risks, such as mortality or early redemption. Deep hedging can be used to more accurately model these variables.
Higgins says his firm’s research found that for natural gas storage, deep hedging lowered transaction costs by 50–80% compared to the standard risk-neutral hedges, depending on the market structure, while the standard deviation of P&L was 50–90% smaller.
Other quants say these sort of techniques could mark a frontier in pricing and hedging derivatives.
“There is no fundamental physical law that governs risk factor dynamics,” says Alexei Kondratyev, a managing director at Standard Chartered in London.
“Our best hope is to take the vast amount of available data and analyse it in its entirety, without imposing any convenient mathematical models. This is why keeping the hedging model free and Greek free is such an appealing proposition.”
This is not to say the Greeks will be consigned to the scrapheap. Buehler says they still form the backbone of risk limit-setting for books that are automatically hedged.
“We still use the Greeks as a risk control. We have limits on vega, we have limits on term structure and so on. But in terms of the hedging decision, it’s no longer used,” he says.
Deep Hedging에 참여한 교수가 Josef Teichmann입니다. 교수가 운용하는 Teaching을 보면 Deep Hedging과 관련한 교육과정 자료가 있습니다.
- Lecture 1 (Introduction, Universal Approximation by shallow networks, one string of arguments for depth): Lecture 1 as iPython notebook, or Lecture 1 as html file and some training data (should be unpacked, then store the files in the folder of the notebook). For the exercises see also Function approximation with linear models and neural network from Tirthajyoti Sarkar's github resources.
- Lecture 2 (neural ordinary differential equations, backpropagation, expressiveness by randomness): Lecture 2 as iPython notebook, or Lecture 2 as html file and some data-file. For the exercises just write a two-hidden layer network by hand with backpropagation by hand following the code in the notebook.
- Lecture 3 (Deep Hedging without transaction costs): Lecture 3 as iPython notebook, or Lecture 2 as html file. For the exercises just get acquainted with the code of the Deep Hedging.
- Lecture 4 (Deep Portfolio Optimization under transaction costs): Lecture 4 as iPython notebook, or Lecture 4 as html file. Some general thoughts on training you can find as iPython notebook, or as html file here. A very short Keras implementation of deep portfolio optimization with transaction costs you can find at as iPython notebook, or as html file.
- Lecture 5 (Deep Simulation): Lecture 5 as iPython notebook, or Lecture 5 as html file. These notes include an introduction on iterated integrals of controls and on the Johnson-Lindenstrauss Lemma as well as code on 'learning' unknown S(P)DEs and simultating real markets.
- Lecture 6 (Deep Calibration): Lecture 6 as iPython notebook, or Lecture 6 as html file. Code is available for calibration via learning a pricing functional as iPython notebook, or as html file, and for a learning the local volatility function in a local stochastic volatility model as iPython notebook, or as html file.
- Lecture 7 (Deep Reinforcement Learning): a short theoretical introduction to concepts of reinforcement learning as html file or as iPython notebook. Some code is added.
- Questions for the exam: a list of questions for the exam as html file or as iPython notebook is added.
그리고 JP Morgan의 퀀트인 Dr. Hans Buehler의 홈페이지는 http://www.quantitative-research.de/입니다.
참고로 JP Morgan이 발행한 기계학습 및 빅데이타 안내서을 쓸 때 저작권때문에 올리지 못한 보고서를 올립니다. 출처는 Big Data and AI Strategies입니다.
[gview file="http://smallake.kr/wp-content/uploads/2019/10/BIG-Data_AI-JPMmay2017.pdf"]
Posted from my blog with SteemPress : http://smallake.kr/?p=28006