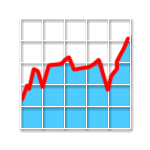
By a News Reporter-Staff News Editor at Investment Weekly News -- Investigators discuss new findings in Intelligent Data Analysis. According to news reporting originating from Selangor Darul, Malaysia, by VerticalNews correspondents, research stated, “Stock price prediction has been an attractive research domain for both investors and computer scientists for more than a decade. Reaction prediction to the stock market, especially based on released financial news articles and published stock prices, still poses a great challenge to researchers because the prediction accuracy is relatively low.”
Our news editors obtained a quote from the research from the University of Kebangsaan, “For prediction purposes, linear regression is a popular method. Statistical metrics, such as the Document Frequency (DF), term frequency-invert document frequency (TF-IDF) and information gain (IG), are used for feature selection to extract the most expressive features to reduce the high dimensionality of the data. However, the effectivenesses of the available metrics have not been explored in identifying important financial feature representations that have dependable and strong relations with the stock price. The objective of this study are (i) to investigate the performance of five statistical metrics, namely, DF, TF-IDF, IG, Chi-square Statistics (Chi-Sqr) and occurrence in identifying important features that can represent the news and have a strong relationship with the stock price; (ii) to introduce feedback variables, namely, the prediction accuracy (PA), directional accuracy (DA) and closeness accuracy (CA), to capture the interaction between the released news and the published stock prices; and (iii) to introduce a prediction model that integrates features from financial news and a stock price value series based on a 20-minute time lag using linear regression. The experiment used the ELR-BoW method to build a number of 330 datasets with five statistical metrics to select different feature sizes of 50, 100, 150, 200, 250, 300, 400, 500, 600, 700 and 800. The performance of ELR-BoW is observed based on three parameters, namely, PA, DA and CA, and is compared against Naive Bayes (NB) as the benchmark approach and the Support Vector Machine (SVM). The proposed ELR-BoW-SVM obtained a higher accuracy compared to ELR-BoW-NB, where the best feedback measure is PA, which has an F-measure value of 0.842. In addition, the best number of features is 300 features and using document frequency DF statistical metric. The identification of the top feature representations for financial news is highly promising for automatic news processing for stock prediction.”
According to the news editors, the research concluded: “This study demonstrates that the identification of the top feature representations for financial news is highly promising for news article processing in stock prediction.”
For more information on this research see: An enhanced feature representation based on linear regression model for stock market prediction. Intelligent Data Analysis , 2018;22(1):45-76. Intelligent Data Analysis can be contacted at: Ios Press, Nieuwe Hemweg 6B, 1013 Bg Amsterdam, Netherlands.
The news editors report that additional information may be obtained by contacting H.A.K. Ihlayyel, Univ Kebangsaan Malaysia, Fac Informat Sci & Technol, Bangi 43600, Selangor Darul, Malaysia. Additional authors for this research include N.M. Sharef, M.Z.A. Nazri and A. Abu Bakar.
The direct object identifier (DOI) for that additional information is: https://doi.org/10.3233/IDA-163316. This DOI is a link to an online electronic document that is either free or for purchase, and can be your direct source for a journal article and its citation.
Our reports deliver fact-based news of research and discoveries from around the world. Copyright 2018, NewsRx LLC
CITATION: (2018-04-14), New Intelligent Data Analysis Findings from University of Kebangsaan Described (An enhanced feature representation based on linear regression model for stock market prediction), Investment Weekly News, 499, ISSN: 1945-8185, BUTTER® ID: 015455795
From the newsletter Investment Weekly News.
https://www.newsrx.com/Butter/#!Search:a=15455795
This is a NewsRx® article created by NewsRx® and posted by NewsRx®. As proof that we are NewsRx® posting NewsRx® content, we have added a link to this steemit page on our main corporate website. The link is at the bottom left under "site links" at https://www.newsrx.com/NewsRxCorp/.
We have been in business for more than 20 years and our full contact information is available on our main corporate website.
We only upvote our posts after at least one other user has upvoted the article to increase the curation awards of upvoters.
NewsRx® offers 195 weekly newsletters providing comprehensive information on all professional topics, ranging from health, pharma and life science to business, tech, energy, law, and finance. Our newsletters report only the most relevant and authoritative information from qualified sources.
Hi! I am a robot. I just upvoted you! I found similar content that readers might be interested in:
https://content.iospress.com/journals/intelligent-data-analysis/22/1
Downvoting a post can decrease pending rewards and make it less visible. Common reasons:
Submit