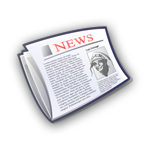
By a News Reporter-Staff News Editor at Journal of Robotics & Machine Learning -- Data detailed on Pattern Analysis have been presented. According to news reporting originating in Curitiba, Brazil, by VerticalNews journalists, research stated, “The detection of anomalous behaviors in automated video surveillance is a recurrent topic in recent computer vision research. Depending on the application field, anomalies can present different characteristics and challenges.”
Financial support for this research came from CNPq.
The news reporters obtained a quote from the research from the Federal University of Technology, “Convolutional Neural Networks have achieved the state-of-the-art performance for object recognition in recent years, since they learn features automatically during the training process. From the anomaly detection perspective, the Convolutional Autoencoder (CAE) is an interesting choice, since it captures the 2D structure in image sequences during the learning process. This work uses a CAE in the anomaly detection context, by applying the reconstruction error of each frame as an anomaly score. By exploring the CAE architecture, we also propose a method for aggregating high-level spatial and temporal features with the input frames and investigate how they affect the CAE performance. An easy-to-use measure of video spatial complexity was devised and correlated with the classification performance of the CAE. The proposed methods were evaluated by means of several experiments with public-domain datasets.”
According to the news reporters, the research concluded: “The promising results support further research in this area.”
For more information on this research see: A study of deep convolutional auto-encoders for anomaly detection in videos. Pattern Recognition Letters , 2018;105():13-22. Pattern Recognition Letters can be contacted at: Elsevier Science Bv, PO Box 211, 1000 Ae Amsterdam, Netherlands. (Elsevier - www.elsevier.com; Pattern Recognition Letters - http://www.journals.elsevier.com/pattern-recognition-letters/)
Our news correspondents report that additional information may be obtained by contacting M. Ribeiro, Fed Univ Technol Parana UTFPR, BR-80230901 Curitiba, PR, Brazil. Additional authors for this research include A.E. Lazzaretti and H.S. Lopes.
The direct object identifier (DOI) for that additional information is: https://doi.org/10.1016/j.patrec.2017.07.016. This DOI is a link to an online electronic document that is either free or for purchase, and can be your direct source for a journal article and its citation.
Our reports deliver fact-based news of research and discoveries from around the world. Copyright 2018, NewsRx LLC
CITATION: (2018-04-23), Researchers at Federal University of Technology Target Pattern Analysis (A study of deep convolutional auto-encoders for anomaly detection in videos), Journal of Robotics & Machine Learning, 296, ISSN: 1944-186X, BUTTER® ID: 015548130
From the newsletter Journal of Robotics & Machine Learning.
https://www.newsrx.com/Butter/#!Search:a=15548130
This is a NewsRx® article created by NewsRx® and posted by NewsRx®. As proof that we are NewsRx® posting NewsRx® content, we have added a link to this steemit page on our main corporate website. The link is at the bottom left under "site links" at https://www.newsrx.com/NewsRxCorp/.
We have been in business for more than 20 years and our full contact information is available on our main corporate website.
We only upvote our posts after at least one other user has upvoted the article to increase the curation awards of upvoters.
NewsRx® offers 195 weekly newsletters providing comprehensive information on all professional topics, ranging from health, pharma and life science to business, tech, energy, law, and finance. Our newsletters report only the most relevant and authoritative information from qualified sources.