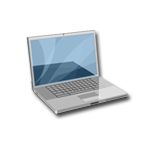
By a News Reporter-Staff News Editor at Computers, Networks & Communications -- New research on Information Technology - Information Theory is the subject of a report. According to news reporting out of Heilongjiang, People’s Republic of China, by VerticalNews editors, research stated, “Usually, it is very difficult to determine the exact distribution for a test statistic. In this paper, asymptotic distributions of locally most powerful invariant test for independence of complex Gaussian vectors are developed.”
Financial supporters for this research include National Natural Science Foundation of China, Natural Science Foundation of Guangdong Province, Foundation of Shenzhen Government.
Our news journalists obtained a quote from the research from the Harbin Institute of Technology, “In particular, its cumulative distribution function (CDF) under the null hypothesis is approximated by a function of chi-squared CDFs. Moreover, the CDF corresponding to the non-null distribution is expressed in terms of non-central chi-squared CDFs for close hypothesis, and Gaussian CDF as well as its derivatives for far hypothesis. The results turn out to be very accurate in terms of fitting their empirical counterparts. Closed-form expression for the detection threshold is also provided.”
According to the news editors, the research concluded: “Numerical results are presented to validate our theoretical findings.”
For more information on this research see: Approximate Asymptotic Distribution of Locally Most Powerful Invariant Test for Independence: Complex Case. IEEE Transactions on Information Theory , 2018;64(3):1784-1799. IEEE Transactions on Information Theory can be contacted at: Ieee-Inst Electrical Electronics Engineers Inc, 445 Hoes Lane, Piscataway, NJ 08855-4141, USA. (Institute of Electrical and Electronics Engineers - http://www.ieee.org/; IEEE Transactions on Information Theory - http://ieeexplore.ieee.org/xpl/RecentIssue.jsp?punumber=18)
Our news journalists report that additional information may be obtained by contacting Y.H. Xiao, Harbin Inst Technol, Dept. of Elect Engn, Harbin 150001, Heilongjiang, People’s Republic of China. Additional authors for this research include L. Huang, J.H. Xie and H.C. So.
The direct object identifier (DOI) for that additional information is: https://doi.org/10.1109/TIT.2017.2669021. This DOI is a link to an online electronic document that is either free or for purchase, and can be your direct source for a journal article and its citation.
Our reports deliver fact-based news of research and discoveries from around the world. Copyright 2018, NewsRx LLC
CITATION: (2018-03-22), New Findings Reported from Harbin Institute of Technology Describe Advances in Information Theory (Approximate Asymptotic Distribution of Locally Most Powerful Invariant Test for Independence: Complex Case), Computers, Networks & Communications, 336, ISSN: 1944-1568, BUTTER® ID: 015350489
From the newsletter Computers, Networks & Communications.
https://www.newsrx.com/Butter/#!Search:a=15350489
This is a NewsRx® article created by NewsRx® and posted by NewsRx®. As proof that we are NewsRx® posting NewsRx® content, we have added a link to this steemit page on our main corporate website. The link is at the bottom left under "site links" at https://www.newsrx.com/NewsRxCorp/.
We have been in business for more than 20 years and our full contact information is available on our main corporate website.
We only upvote our posts after at least one other user has upvoted the article to increase the curation awards of upvoters.
NewsRx® offers 195 weekly newsletters providing comprehensive information on all professional topics, ranging from health, pharma and life science to business, tech, energy, law, and finance. Our newsletters report only the most relevant and authoritative information from qualified sources.