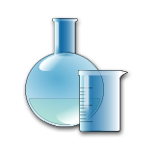
By a News Reporter-Staff News Editor at Life Science Weekly -- Researchers detail new data in Life Science Research. According to news reporting out of Jiangsu, People’s Republic of China, by NewsRx editors, research stated, “The traditional taxi prediction methods model the taxi trajectory as a sequence of spatial points. It cannot represent two-dimensional spatial relationships between trajectory points.”
Financial support for this research came from Fundamental Research Funds for the Central Universities.
Our news journalists obtained a quote from the research from the China University of Mining and Technology, “Therefore, many methods transform the taxi GPS trajectory into a two-dimensional image, and express the spatial correlations by trajectory image. However, the trajectory image may have noise and sparsity according to trajectory data characteristics. So, we import image frequency domain processing to taxi destination prediction to reduce noise and sparsity, then propose multi-features taxi destination prediction with frequency domain processing (MTDP-FD) method. Firstly, we transform the spatial domain trajectory image into frequency-domain representation by fast Fourier transform and reduce the noise of the trajectory images. Convolutional Neural Network (CNN) is adapted to extract the deep features from the processed trajectory image as CNN has a significant learning ability to images. Recurrent Neural Network (RNN) is adapted to predict the taxi destination as multiple hidden layers of RNN can store dependencies between input data to achieve better prediction. The deep features of the trajectory images are combined with trajectory metadata, trajectory data to act as the input to RNN.”
According to the news editors, the research concluded: “The experiments based on the taxi trajectory dataset of Porto show that the average distance error of MTDP-FD is reduced by 0.14km compared with the existing methods, and the GTOHL is the best combination of data and features to improve the prediction accuracy.”
For more information on this research see: Multi-features taxi destination prediction with frequency domain processing. Plos One , 2018;13(3):e0194629. (Public Library of Science - www.plos.org; Plos One - www.plosone.org)
Our news journalists report that additional information may be obtained by contacting L. Zhang, School of Computer Science and Technology, China University of Mining and Technology, Xuzhou, Jiangsu, People’s Republic of China. Additional authors for this research include G. Zhang, Z. Liang and E.F Ozioko.
The direct object identifier (DOI) for that additional information is: https://doi.org/10.1371/journal.pone.0194629. This DOI is a link to an online electronic document that is either free or for purchase, and can be your direct source for a journal article and its citation.
Our reports deliver fact-based news of research and discoveries from around the world. Copyright 2018, NewsRx LLC
CITATION: (2018-04-10), Researchers from China University of Mining and Technology Detail Findings in Life Science Research (Multi-features taxi destination prediction with frequency domain processing), Life Science Weekly, 3650, ISSN: 1552-2474, BUTTER® ID: 015450769
From the newsletter Life Science Weekly.
https://www.newsrx.com/Butter/#!Search:a=15450769
This is a NewsRx® article created by NewsRx® and posted by NewsRx®. As proof that we are NewsRx® posting NewsRx® content, we have added a link to this steemit page on our main corporate website. The link is at the bottom left under "site links" at https://www.newsrx.com/NewsRxCorp/.
We have been in business for more than 20 years and our full contact information is available on our main corporate website.
We only upvote our posts after at least one other user has upvoted the article to increase the curation awards of upvoters.
NewsRx® offers 195 weekly newsletters providing comprehensive information on all professional topics, ranging from health, pharma and life science to business, tech, energy, law, and finance. Our newsletters report only the most relevant and authoritative information from qualified sources.