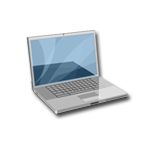
By a News Reporter-Staff News Editor at Computer Weekly News -- Current study results on Computers - Computer Programming have been published. According to news reporting from Lawrence, Kansas, by VerticalNews journalists, research stated, “Constructing distributed representations for words through neural language models and using the resulting vector spaces for analysis has become a crucial component of natural language processing (NLP). However, despite their widespread application, little is known about the structure and properties of these spaces.”
Financial supporters for this research include NSF: CGV, NSF:IIP, NSF:ACI, DOE/SciDAC, CCMSC, PIPER, Department of Energy, National Nuclear Security Administration.
The news correspondents obtained a quote from the research from Lawrence Livermore National Laboratory, “To gain insights into the relationship between words, the NLP community has begun to adapt high-dimensional visualization techniques. In particular, researchers commonly use t-distributed stochastic neighbor embeddings (t-SNE) and principal component analysis (PCA) to create two-dimensional embeddings for assessing the overall structure and exploring linear relationships (e.g., word analogies), respectively. Unfortunately, these techniques often produce mediocre or even misleading results and cannot address domain-specific visualization challenges that are crucial for understanding semantic relationships in word embeddings. Here, we introduce new embedding techniques for visualizing semantic and syntactic analogies, and the corresponding tests to determine whether the resulting views capture salient structures. Additionally, we introduce two novel views for a comprehensive study of analogy relationships. Finally, we augment t-SNE embeddings to convey uncertainty information in order to allow a reliable interpretation.”
According to the news reporters, the research concluded: “Combined, the different views address a number of domain-specific tasks difficult to solve with existing tools.”
For more information on this research see: Visual Exploration of Semantic Relationships in Neural Word Embeddings. IEEE Transactions on Visualization and Computer Graphics , 2018;24(1):553-562. IEEE Transactions on Visualization and Computer Graphics can be contacted at: Ieee Computer Soc, 10662 Los Vaqueros Circle, PO Box 3014, Los Alamitos, CA 90720-1314, USA. (Institute of Electrical and Electronics Engineers - http://www.ieee.org/; IEEE Transactions on Visualization and Computer Graphics - http://ieeexplore.ieee.org/xpl/RecentIssue.jsp?punumber=2945)
Our news journalists report that additional information may be obtained by contacting S.S. Liu, Lawrence Livermore Natl Lab, Lawrence, KS 94550, United States. Additional authors for this research include P.T. Bremer, J.J. Thiagarajan, V. Srikumar, B. Wang, Y. Livnat and V. Pascucci.
The direct object identifier (DOI) for that additional information is: https://doi.org/10.1109/TVCG.2017.2745141. This DOI is a link to an online electronic document that is either free or for purchase, and can be your direct source for a journal article and its citation.
Our reports deliver fact-based news of research and discoveries from around the world. Copyright 2018, NewsRx LLC
CITATION: (2018-01-10), Reports Outline Computer Programming Study Results from Lawrence Livermore National Laboratory (Visual Exploration of Semantic Relationships in Neural Word Embeddings), Computer Weekly News, 532, ISSN: 1944-1606, BUTTER® ID: 014933874
From the newsletter Computer Weekly News.
https://www.newsrx.com/Butter/#!Search:a=14933874
NewsRx® offers 195 weekly newsletters providing comprehensive information on all professional topics, ranging from health, pharma and life science to business, tech, energy, law, and finance. Our newsletters report only the most relevant and authoritative information from qualified sources.
Hi! I am a robot. I just upvoted you! I found similar content that readers might be interested in:
Downvoting a post can decrease pending rewards and make it less visible. Common reasons:
Submit