The Paper
The paper that caught my eye today is a paper discussing the application of machine learning to the diagnosis of Schizophrenia by looking at electroencephalogram (EEG) data. The article title is Auditory prediction errors as individual biomarkers of schizophrenia, and is written by a group of mainly Australian researchers, including two colleagues of mine, Natasha Matthews and Pat Michie.
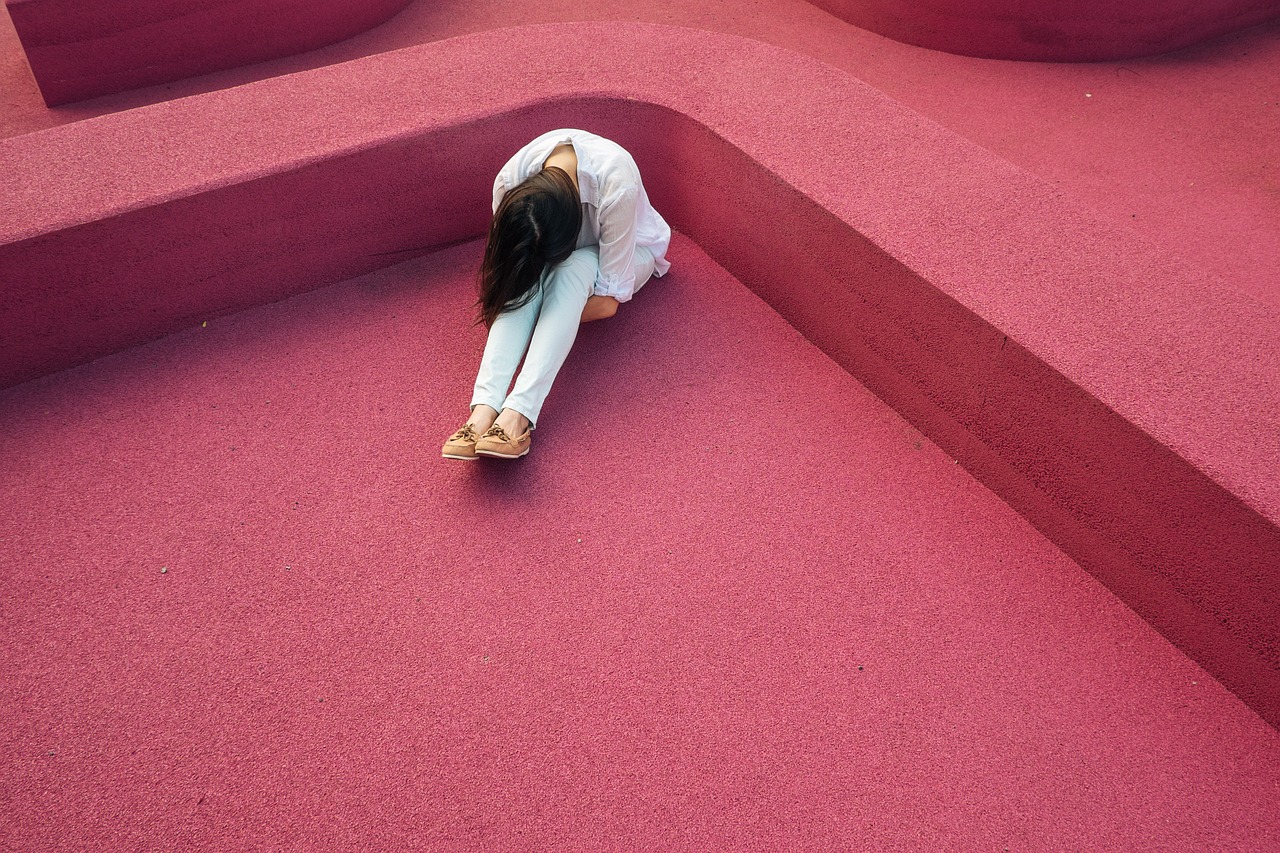
Background information
Since it's first descriptions by physicians and psychiatrists in the late 1800's and the coining of the term schizophrenia in 1908 by Eugen Bleuler the precise definition has been in flux. The current (DSM-5) guidelines for diagnosis specify at least 6 months of ongoing psychopathology, including some (but not necessarily all) of the following aspects:
- Delusions (Thinking people are "out to get you", thinking you are a famous figure, believing your thoughts and actions are being controlled by an outside force.)
- Hallucinations (Sounds or sensations experienced as real that only happen in the persons mind, in schizophrenia these are usually attributed meaning)
- Disorganised Speech (Trouble concentrating or maintaining a train of thought, speaking incoherently)
- Disorganised Behaviour (Bizarre, purposeless behaviours, lack of inhibition and impulse control)
- Negative Symptoms (Lack of motivation, general cognitive decline, unawareness of the environment)
As these symptoms can vary in degree and aspect, and the fact that a full diagnosis requires assessment over a long time period by a highly qualified individual then the cost of service provision is very expensive. This, combined with the struggle to get people suffering from psychotic symptoms or lack of motivations from seeing said professional means the ability to diagnose (or at least assist diagnosis) with an automated tool would be of great value, freeing up the time for professionals to focus on interventions and getting treatment earlier.
Motivation for the study
The diagnosis of schizophrenia is based on self report measures, and while previous studies suggest the validity of schizophrenia diagnoses is approximately 90%, the fact that the judgement is necessarily subjective in the end means the discovery of a reliable biomarker to help inform these decisions would be of great value. There have been several biomarker alternatives proposed for schizophrenia over the years focussing on genetic, MRI and EEG measures, and one of the more robust results within the schizophrenia population is the reduction in mismatch negativity (MMN) EEG waveforms, which is the brains response to a surprising (deviant) stimulus after the build up of a prediction based on a regular or common (standard) stimulus. The predominant form of paradigm that elicits this response is an auditory task (an oddball task)
The goals of the study were to develop a predictive model that aids in the diagnosis of schizophrenia, assessing the performance of different oddball tasks, classification algorithms and analysis strategies.
How the study was performed
A little over 20 schizophrenia patients and matched controls were recruited to participate in the study, with patients diagnoses being verified by way of the Diagnostic Interview for Psychosis and controls were verified to be free of significant psychopathology using the same tool.
Three different oddball (MMN eliciting) paradigms were run for all participants. In one the deviant (oddball) sounds were longer in duration, the second had deviants with a short gap in the middle of the sound and the final task had deviants where the sound presented to one ear was slightly behind the other ear (making the sound appear to be coming from the left or right). Each of these tasks have historically shown strong results in schizophrenia populations.
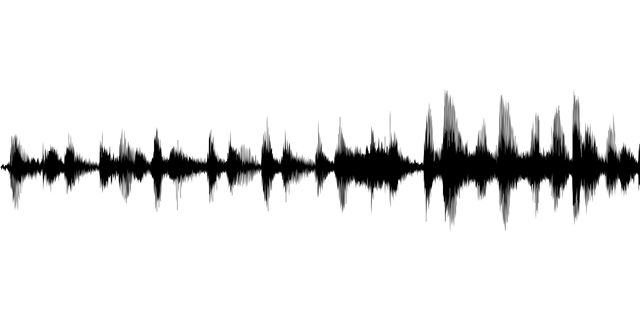
The extracted EEG features and clinical measures that were acquired were then fed into a number of machine learning algorithms to test for binary classification of subjects between the Schizophrenia/Control groups as well as investigating quantifiable relationships between neuronal responses and the severity of symptoms.
Results of the study
The classification of subjects into the schizophrenia/control groups was able to be performed with slightly over 80% accuracy - yielding better results than similar approaches of machine learning to classification of schizophrenia in the past - perhaps due to the fact that the whole spatio-temporal EEG signal was input into the classifiers, as opposed to just certain extracted features. There was a higher accuracy of 90% reported on a previous study, however they used fMRI data for there classification, which is an expensive diagnostic tool.
However whilst the results are promising, one fact that is raised is that schizophrenia is not a binary condition (otherwise healthy controls may have mild schizotypal symptoms, and in general symptoms vary a lot in terms of expression and severity), therefore the training of a binary classifier raises some concerns.
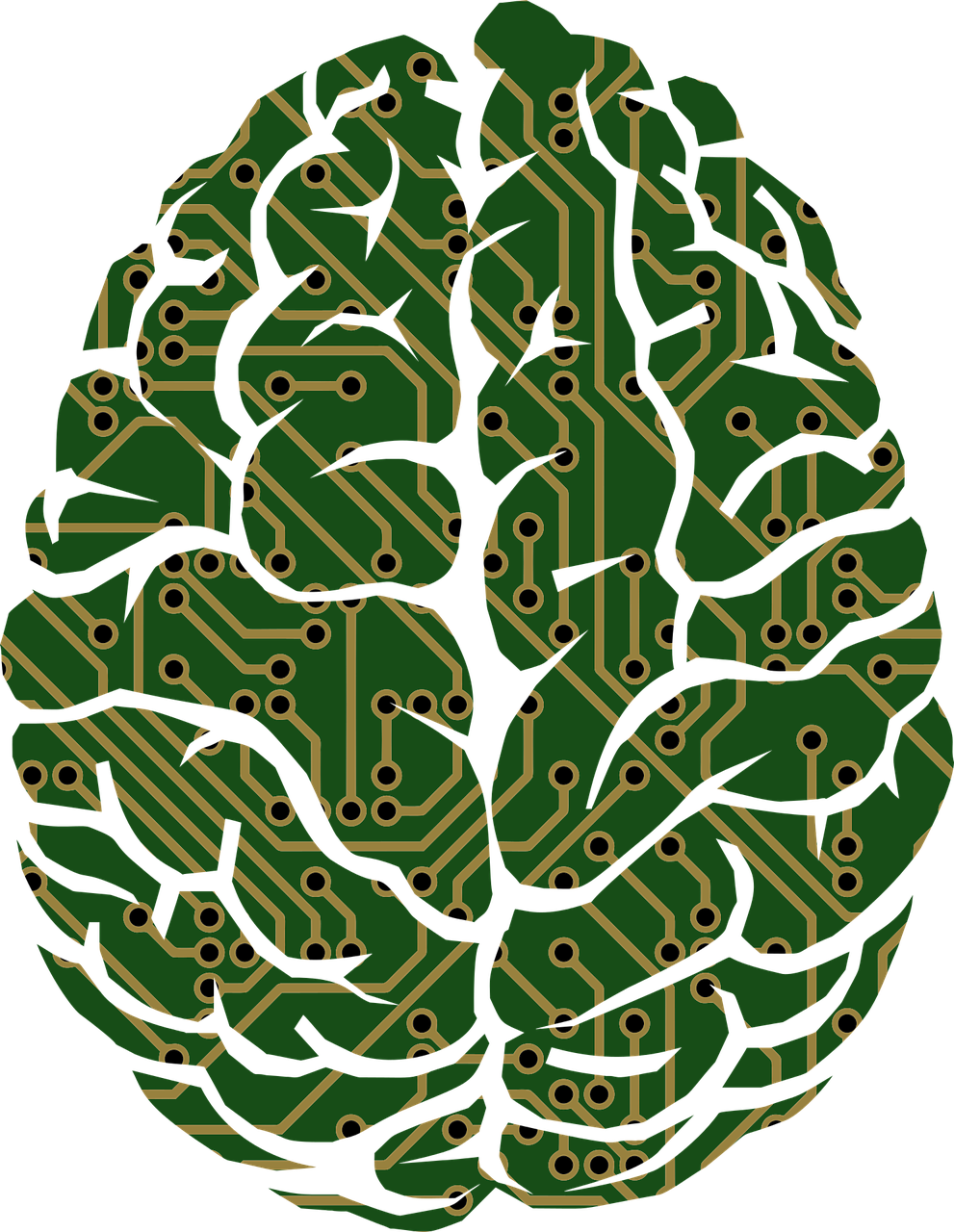
My Takeaway Message
Whilst this study provides some incentive to do more research, especially in a much larger dataset of people who have just been diagnosed (before long term treatment), it does not provide a definitive solution to the problem of diagnosis in schizophrenia. Another aspect I would be wary of is that MMN reduction is not limited to people with Schizophrenia, so extensive research including these conditions would probably be appropriate to tease out any schizophrenia specific features (if such exist).
This is a great summary post @gjcooper. I enjoyed reading it. You did a great job taking all the science in the original journal article and turning it into plain English for us lay people to understand.
I see you are new to steemit. Welcome! We have a pretty strong Team Australia community going here on steemit to support each other. If you'd like to be part of it, then please check out this link for the instructions on how to join https://steemit.com/teamaustralia/@choogirl/team-australia-new-recruits-update-21-11-17-and-the-steemit-growth-forum
Downvoting a post can decrease pending rewards and make it less visible. Common reasons:
Submit
Thanks for your kind words, I'll have a more in depth read of that link when I get a chance as it looks like an oipportunity I'd love to follow up on.
Downvoting a post can decrease pending rewards and make it less visible. Common reasons:
Submit
Congratulations @gjcooper! You received a personal award!
You can view your badges on your Steem Board and compare to others on the Steem Ranking
Do not miss the last post from @steemitboard:
Vote for @Steemitboard as a witness to get one more award and increased upvotes!
Downvoting a post can decrease pending rewards and make it less visible. Common reasons:
Submit
Congratulations @gjcooper, this post is the most rewarded post (based on pending payouts) in the last 12 hours written by a Dust account holder (accounts that hold between 0 and 0.01 Mega Vests). The total number of posts by Dust account holders during this period was 4976 and the total pending payments to posts in this category was $692.71. To see the full list of highest paid posts across all accounts categories, click here.
If you do not wish to receive these messages in future, please reply stop to this comment.
Downvoting a post can decrease pending rewards and make it less visible. Common reasons:
Submit
Congratulations, your post received one of the top 10 most powerful upvotes in the last 12 hours. You received an upvote from @hendrikdegrote valued at 103.25 SBD, based on the pending payout at the time the data was extracted.
If you do not wish to receive these messages in future, reply with the word "stop".
Downvoting a post can decrease pending rewards and make it less visible. Common reasons:
Submit
You started early on with "coining of the term".
Are you giving forewarning of a SchizoCoin?
Downvoting a post can decrease pending rewards and make it less visible. Common reasons:
Submit
Congratulations @gjcooper! You have received a personal award!
Click on the badge to view your Board of Honor.
Do not miss the last post from @steemitboard:
Downvoting a post can decrease pending rewards and make it less visible. Common reasons:
Submit
everyone comments on Steem for the win
Downvoting a post can decrease pending rewards and make it less visible. Common reasons:
Submit
study is the main part of life
Downvoting a post can decrease pending rewards and make it less visible. Common reasons:
Submit
Machines and automated process can accomplish so much of what was formerly thought of as human work.
Downvoting a post can decrease pending rewards and make it less visible. Common reasons:
Submit
I agree, but I like to think of automated processes taking up tasks that are either boring and repetitive or are helped by being able to sift huge amounts of data. In this way they can augment us mere humans in order to increase our productivity.
I think it is a long (extremely long) time before we entrust things like the diagnosis of psychiatric disorders solely to the province of a machine.
Downvoting a post can decrease pending rewards and make it less visible. Common reasons:
Submit
Hey come and give a shot and see how much you know about deep-learning by explaining the joke and at the same time promote learning AI.
Downvoting a post can decrease pending rewards and make it less visible. Common reasons:
Submit
Congratulations @gjcooper! You have completed some achievement on Steemit and have been rewarded with new badge(s) :
Click on any badge to view your own Board of Honor on SteemitBoard.
For more information about SteemitBoard, click here
If you no longer want to receive notifications, reply to this comment with the word
STOP
Downvoting a post can decrease pending rewards and make it less visible. Common reasons:
Submit
Nice post... Hopefully they will find a better treatment in the near future.
Downvoting a post can decrease pending rewards and make it less visible. Common reasons:
Submit
Unfortunately it has been very slow going, but prognosis (how sick someone gets) is improved the earlier this and other disorders are detected. Hopefully with earlier diagnosis using tools perhaps like this we can help people earlier and keep them as happy and relatively healthy members of our communities.
Downvoting a post can decrease pending rewards and make it less visible. Common reasons:
Submit