"In the previous year, we have observed the meteoric rise of Artificial Intelligence and Machine Learning. From huge platform providers to early-stage firms, AI and ML have grown to be the key concentration areas."
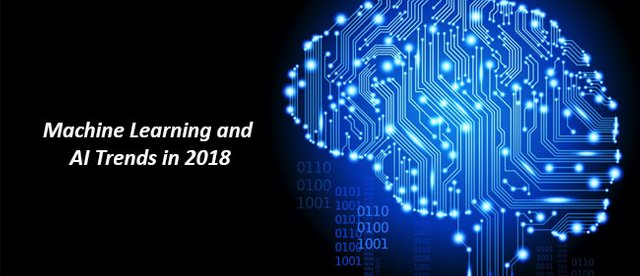
Image Source
In the previous year, we have observed the meteoric rise of Artificial Intelligence and Machine Learning. From huge platform providers to early-stage firms, AI and ML have grown to be the key concentration areas. VCs rained vast amounts of money in financing AI-associated startups. Platform businesses elevated their R & D finances to speed up study in AI & ML domains. The amount of web-based classes providing self-paced learning has to strike the rooftop. Lastly, there is absolutely no solitary sector vertical that is not influenced by AI.
Although it is now a cliché, democratizing machine learning has brought away in 2017. Amazon, Apple, IBM, Google, Facebook, and Microsoft are contending with one another to create ML available to programmers. The accessibility of resources and frameworks tripled within just twelve months. 2017 also noticed the start of AI infusion in business apps.
Together with the buzz at its maximum, what is in store for AI and ML in this year?
Key Machine Learning Trends
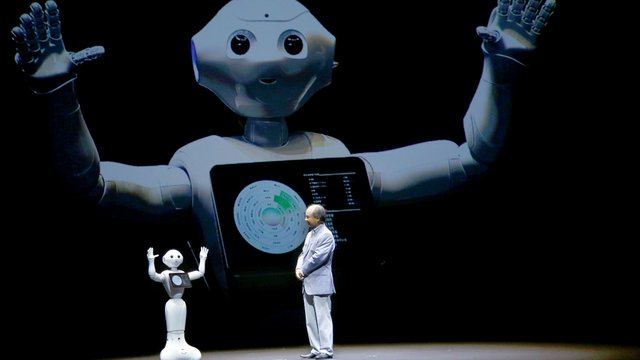
Image Source
Listed below are about three important developments for 2018 which will get AI and ML to a higher level:
1. Inference at the Edge
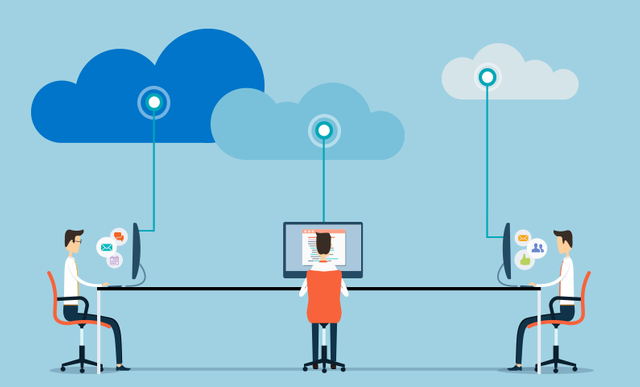
Image Source
Edge computing requires computer nearer to the apps. Each and every edge area computes the general cloud by revealing a suitable pair of solutions and endpoints that the apps can take in. It really is good to go to change business facilities.
The edge computing coating reveals compute, safe-keeping, and network solutions to programmers. Usually, edge computing operates on the top of a constrained structure that might not be effective ample to perform VMs or containers. Here is where serverless takes on an important role in supplying the compute solutions.
Right after virtualization and containerization, serverless is growing as being the following influx of computer providers. Functions as a Service (FaaS), a serverless delivery service design, try to streamline the programmer experience by reducing the functional expense in setting up and handling program code.
Machine learning designs that happen to be completely skilled within the cloud are used in the edge for inference. The hefty lifting occurs within the general cloud whilst the enhanced model is applied to the edge. The inference model is subjected to being a functionality used inside the serverless compute atmosphere.
In the event edge computing layer operates on potent equipment able to working storage containers, the ML inference models are packed and used as storage containers.
AWS Deep Lens and Zure IoT Edge are a sample of how inference designs are used in the edge. AWS Deep Lens runs ML design written in Python being a Lambda functionality. In Azure IoT Edge, the ML components are packed as storage units and forced towards the edge layer.
Machine learning will end up the true secret driver for accelerating the adoption of edge computing.
2. DevOps for Data Science
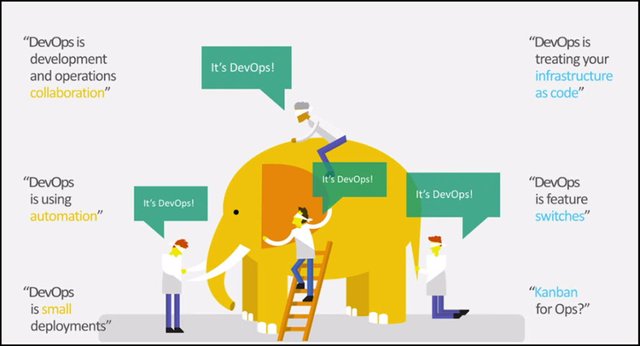
Image Source
A data science is described as a person who seems to be much better in statistics than the average developer along with a far better programmer than a typical statistician. Data scientists squarely concentrate on discovering concealed designs in information sets. They utilize verified statistical designs to contemporary information sets to fix firm difficulties.
Even though information researchers take care of Python, R and Julia to produce machine learning designs, they are certainly not outfitted to handle the facilities and surroundings necessary for building and setting up ML designs. Through the growth stage, ML designs will likely e relocated back and forth among local development surroundings and cloud-based training environments in which GPU-based VMs are used for scale. Data scientists require a straightforward process to execute the roundtrip in between local environment and cloud-based atmosphere.
In the end, a skilled design is an additional bit of program code which needs to be taken care of like every other quest – crucial app. They should be dependably used in safe, readily available, scalable, and trustworthy conditions.
In the training and inference, there exists a substantial amount of facilities plumbing that is required by data scientists. This plumbing consists of establishing the proper improvement surroundings, packaging the program code as compartment pictures, scaling the storage containers throughout the coaching and inference, versioning current models, configuring a pipeline to improve models with more recent versions smoothly, and several other common DevOps jobs.
As data science gets to be well-known, DevOps for data science grows more significantly. This year will see a fully developed and sleek DevOps procedures solely identified for data science.
Amazon Company SageMaker and Azure ML Workbench are early signs of this crazy, the two platforms pinpoint the DevOps part of data science.
3. Artificial Intelligence for IT Operations
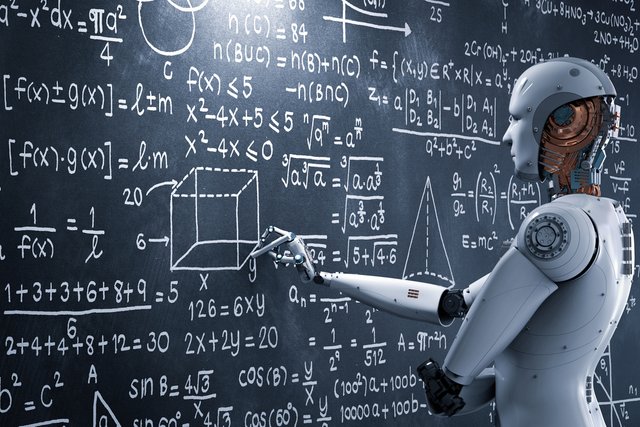
Image Source
Present day programs and facilities are producing log information that is certainly taken for indexing, looking, and analytics. The enormous data sets extracted from the equipment, operating systems, hosting server software program and application software program could be aggregated and linked to locate insights and designs. When machine learning models are placed on these datasets, IT procedures change from simply being reactive to predictive.
When the strength of AI is used to procedures, it is going to change the way in which facilities are handled.
The use of ML and AI in Information Technology operations and DevOps will provide knowledge to companies. It can help the ops groups to carry out exact and precise cause evaluation. Sophisticated models will help avoid interruption and failure to IT via predictive statistics. Invasion recognition could be augmented with ML for improved safety. There are lots of situations in which the use of ML to IT will result in intelligent procedures.
Amazon Macie and Azure Log Analytics are earlier samples of AIOps. Aside from AWS and Azure, numerous startups are making an investment in AI-powered Ops.
@OriginalWorks
Downvoting a post can decrease pending rewards and make it less visible. Common reasons:
Submit
The @OriginalWorks bot has determined this post by @ruelrevales to be original material and upvoted it!
To call @OriginalWorks, simply reply to any post with @originalworks or !originalworks in your message!
Downvoting a post can decrease pending rewards and make it less visible. Common reasons:
Submit
I work a lot in ML. DevOps and Edge are big but I feel like the largest area of ML will be IoT and Medicine. These are the things I have personally seen in the industry getting some traction.
Downvoting a post can decrease pending rewards and make it less visible. Common reasons:
Submit
Notes taken. Thank you! ;)
Downvoting a post can decrease pending rewards and make it less visible. Common reasons:
Submit
I hope this year will be a great one for machine learning experts.
Downvoting a post can decrease pending rewards and make it less visible. Common reasons:
Submit
Let's all hope it does.
Downvoting a post can decrease pending rewards and make it less visible. Common reasons:
Submit
The use of AI in different electronics in the present times are awesome.
Downvoting a post can decrease pending rewards and make it less visible. Common reasons:
Submit
Makes me wonder when high tech robots will walk in the streets like normal objects. Hehe
Downvoting a post can decrease pending rewards and make it less visible. Common reasons:
Submit
Great article!
Downvoting a post can decrease pending rewards and make it less visible. Common reasons:
Submit
Thank you for the appreciation! ;)
Downvoting a post can decrease pending rewards and make it less visible. Common reasons:
Submit
It is the time to go with the revolution of AI.
Downvoting a post can decrease pending rewards and make it less visible. Common reasons:
Submit
Even small startups could start now.
Downvoting a post can decrease pending rewards and make it less visible. Common reasons:
Submit
I appreciate your effort in making this article to spread awareness about the effectiveness AI and ML brings into the society at the current times.
Downvoting a post can decrease pending rewards and make it less visible. Common reasons:
Submit
It is a good thing to hear someone appreciating my hours of passion in making articles. More power for all of us! ;)
Downvoting a post can decrease pending rewards and make it less visible. Common reasons:
Submit